Environment & Camera Set Up For Best Facial Recognition and Video Analytics Results
Environment
To ensure good facial recognition and video analytics results, it is important to get the image capture environment set up correctly. Along with the quality of the lens and camera there are external factors such as lighting conditions, angle, and the natural behaviour of people in environment that must be considered carefully to ensure the successful overall design of the facial recognition and video analytics system.
To obtain effective facial recognition, there is usually the need to do one or more of the following tasks:
• Adjust/optimise the position and lenses of existing cameras, where utilised
• Install additional facial recognition cameras, after a careful site survey
• Correctly configure the settings for the Imagus Facial Recognition and video analytics application.
Considerations
Vix Vizion's Imagus Facial Recognition Technology can capture and recognise faces in a non-cooperative environment. This means that we can use faces captured opportunistically from CCTV cameras where subjects are not behaving in a way to cooperate with or explicitly enable a facial image capture. Nevertheless, good matching requires good quality images. The ideal image is of passport quality and deviations from this ideal image may reduce match accuracy to some degree. Capturing passport photo quality is difficult in non-cooperative mode, but by positioning the cameras carefully and choosing good locations, we can achieve excellent results recognising a large proportion of passing faces. The position and setup of the non-cooperative facial recognition cameras can be different from standard surveillance camera installations. The latter are primarily installed to record activity over a wide field of view from a high location for public liability and other business reasons. Attempts to use existing surveillance cameras without some repositioning and adjustment will reduce the effectiveness of the system.
Below is a list of considerations for positioning existing and new cameras to achieve good facial recognition and video analytics performance:
Managing angles for recognition
Facial recognition is best when we use eye-level cameras. Cooperative capture systems like Smart Gates use multiple or moving cameras to achieve eye-level images leading to quite good verification. This is not generally possible in a non-cooperative environment, although a multi-camera system is an important consideration for priority locations such as doorways.
Generally, surveillance cameras are mounted high on the ceiling and look down on the public. This is done for reasons relevant to surveillance to reduce the obscuration of persons in crowds, provide for easy wiring via the dropped ceiling space, reduce the risk of camera vandalism and tampering and allow for the cameras to be placed higher than the tallest person to avoid collision and injury. This is not optimal for facial recognition because it may mean that shorter people may disappear from view before they get close enough to the camera to recognise them.
Look Down or Slant Angle
Figure 1: Good Facial Recognition View Angles
A problem with ceiling mount cameras for non-cooperative facial recognition is that the look down angle - often called the slant angle - can be quite severe for faces close enough to the camera to recognise. Facial recognition performance drops with large downward slant angles and faces can become quickly obscured by foreheads and hats. A significant upward slant angle is much less of a problem for recognition, but eye-level positioning is best of all.
Figure 2: Wide Angle Lens
As there are excellent reasons to keep surveillance cameras mounted high, how do we reduce the look down angle problem for facial recognition? The most straightforward approach is to adjust the lens to be more telephoto (longer focal length) than is the usual practice for surveillance camera installations, which has two advantages. First, it reduces the slant angle, and second, it reduces the rate of growth in image size with decreasing distance from the Camera, reducing motion blur.
Wide-Angle and Telephoto Lenses
Figure 3: Telephoto Lens
The effect on the motion of wide-angle lenses can be seen in many rap music videos. Artists move their faces and hands close to and then further away from the lens, and there is the effect of exaggerated movement. On the other hand, when a telephoto lens is used to film a Formula 1 racing car, it appears that the speeding cars are crawling around the track. Thus, telephoto lenses reduce both slant angle and motion blur. The disadvantage of telephoto lenses in corridor surveillance is that there is more risk of obscuration of shorter people.
Taking advantage of the stadium effect
So how do we address the potential for obscuration of faces in crowds? This was solved in ancient Greece by placing the audience in an amphitheatre or stadium seated at different heights - then everyone could see the play or public performance. Indeed, some SUVs advertise stadium seating, so that children in the rear seats can have unspoiled views of the road. This simple idea also works for non-cooperative facial recognition image capture. There are many natural stadiums in public spaces. These include ramps, stairs, and escalators.
Ramps are the ideal form of the stadium because people tend to look straight ahead when they walk down a ramp, especially if there is a crowd behind them. A well-positioned camera can obtain an unobscured eye-level view of each person as they pass by, and the telephoto lens will reduce apparent motion towards the camera, giving a large sweet spot for facial recognition. Such down ramps are extremely common in many international airports. Often the departures level is above the arrivals level, and the aerobridges connect midway between the two floors (e.g., Hong Kong Airport). Passengers walk down one ramp to board the aircraft, and down another ramp to disembark. Aerobridges can also be a down ramp.
It may seem that stairs and escalators provide a similar opportunity for face capture for facial recognition, however this is rarely the case in practice because people don’t look towards the camera as often, so we may miss face images. On an escalator, many people stand and look sideways at the advertising. Sometimes they are checking their phones. Because they do not need to walk, they do not look straight ahead. The situation is slightly different on staircases. Here the main risk for a person is falling down the stairs, especially if they are carrying luggage. So, most people spend time looking at the staircase and other members of the public to avoid missteps and falls.
Understanding natural human behaviour
People have natural behaviours, and we must use this knowledge when we position facial recognition cameras. It's quite hard to predict human behaviour, so it's important to observe a video of human behaviour before committing to installing a new facial recognition camera. Ideally, we recommend using a mobile phone to record a few minutes of video from prospective camera locations before committing to camera installation. A selfie stick can be used to raise the phone to the correct height so that a short video can be captured. With this simple site survey, we will be able to provide accurate feedback and advice on installation suitability for facial recognition. We may even be able to recognise faces from this feed.
A second recommendation is to install a PTZ camera for facial recognition purposes — even if it is only a temporary install. This will give us the ability to adjust the pan/tilt and zoom remotely for optimal face capture. Once the PTZ is fully adjusted, it could easily be replaced by a cheaper permanent fixed lens system giving the same view.
As a rule, it's hard to predict where a person will be looking if they are not walking or reading something. Generally, if a person is walking purposefully on flat ground or a gentle slope, they will look in their direction of travel. This is especially true if there is a crowd of commuters behind them. In general, if a person is a commuter, they are less likely to be distracted by advertising as they are concentrating on getting to their destination on time. Many commuters travel through shopping centres on their way to and from work and they will have seen all the shops and advertisements many times. Similarly, people crossing the road at an intersection may be considered commuters during the short trip across the road.
The above description of commuters explains why down ramps at airports work so well for facial recognition and video analytics. If a person needs to change the direction of travel, they will point their head in that direction well before they need to turn. If they are going into a doorway or cross-corridor, people tend to look towards the doorway or cross-corridor well before they change direction. They will also try to cut the corner if they can. Even if walking straight ahead, people will look sideways at junctions to avoid a collision with human cross-traffic. This behaviour is quite important because CCTV installers may place their cameras at the intersections of corridors where there is a high risk of people colliding and injuring themselves. This is not ideal to capture faces. On uneven ground or stairs, people will spend time looking at their feet to avoid a misstep. This is another area where CCTV cameras are may be installed for public liability reasons. Once again, such locations are not ideal for facial recognition because people don't look at the camera often enough.
Narrow doorways can be well-suited to facial recognition face capture if the cameras can be mounted low enough to get a good view. A doorway concentrates people into a narrow field of view which is also convenient. Doorways tend to be busy places, people tend to walk through them quickly to avoid blocking other customers. We call such situations a chokepoint, for example, a revolving doorway can help to regulate traffic and guests and tend to move quickly away from the doorway to avoid being caught in the door. Similarly, people tend to look straight ahead when exiting lifts and elevators to avoid being caught by the automatic doors. Multiple overlapping camera fields may easily cover wider doorways. There may be the possibility of mounting cameras on a suspended decorative display in the foyer which will lower camera height which may also help attract attention.
Another possibility for larger corridors is to mount cameras on any narrow pillars in the pedestrian stream. While a low hanging camera is likely to cause injury, most people will avoid running into a concrete pillar. Unlike mounting a camera on a wall, people will walk straight toward pillars with only slight deviations in their path to avoid the obstacle. Even better, they tend to look straight at the pillar to avoid collision. Similarly, we achieve very good face-in-the-crowd facial recognition results using a camera temporarily mounted on a tripod. The crowd walks around the eye-level camera as they file past.
The below figures explain the camera field of view (FOV) using the shopping mall entry door.
Figure 4: General CCTV wide-angle FOV with a door entrance camera enabled
with facial recognition at a shopping mall.
Figure 5: Ideal camera FOV that focuses on the door entrance
for the best facial recognition result.
Attracting people's attention
The main challenge capturing faces in a crowd is that people tend to walk by quite fast. This can cause motion blur affecting facial recognition performance. Ideally, we want the person to slow down or stand still and look at the eye-level camera without asking them to do so. So, we need to find a way to grab and maintain their attention. One method is to take advantage of turnstiles, gates and ticket barriers. For example, commuters slow down to validate tickets. Passengers may look at a card reader/slot, so a camera placed appropriately in the card reader provides for great face capture. Alternatively, for example, two 5 MP CCTV cameras could cover, say, 12 laneways. After the ticketing barrier, there is often a set of information screens that encourage commuters to look up and check the timetable. This is also a good spot to place facial recognition cameras. Note that people tend to stand still and look in one direction for some time when they are reading an information screen.
Point of sales facial recognition
People need to look at the sales assistant when they purchase at a point of sale. Eye level cameras at this location are ideal for facial recognition and video analytics because the person is virtually motionless and looking forward, and there is generally good lighting.
Lighting considerations
High Ceilings
To recognise faces, it is helpful to have high ceilings with soft light from all directions, but mostly from overhead. High ceilings mean that the lighting angle does not vary significantly as the subject moves in the field of view.
Low Ceilings
Low ceilings with halogen spotlights can reduce effective facial recognition. Difficult situations may occur in carparks because of the low ceilings and the sparse sodium and fluorescent lighting. Some problems may be addressed by using additional lighting, adding diffusers to the lights, or positioning the camera carefully.
Entry Doors
Doorways with bright natural light can cause challenges. Natural light from the Sun also changes with the time of day. We recommend surveying the site over various times of day to assess suitability and camera locations for facial recognition. Backlight can also be experienced in situations such as entering a bus or using an ATM. These capture conditions require careful planning and camera positioning. In a typical CCTV environment, a wide-dynamic range camera would usually be recommended. Wide-dynamic range cameras generally capture several shots of the scene at different exposure levels. This creates overexposed and underexposed identical images, which the camera will combine using the most balanced parts of each image. This is good for overall CCTV surveillance but can create blurred imaging for facial recognition. For facial recognition and video analytics we recommend turning wide-dynamic range "off", instead using backlight compensation (BLC) to enable a better quality image.
Additional Notes On-Camera Location and Setup
The following configuration will ensure satisfactory, real-time, video-based facial recognition and video analytics. The Internet, Camera, and Server Configurations below will help guide you through the setup. A sample parts list is provided for the server - configured for an entry-level PC to connect a maximum of 4 cameras. Please note: cameras explicitly used for facial recognition are used to complement not replace any existing CCTV system.
Clarification of Face Detection for Facial Recognition
A camera needs to have a clear, unobstructed view of a person's face for facial recognition. Placement of a camera should be at a chokepoint, such as an entry door. The use of an existing camera from a current public surveillance system may not be ideal for facial recognition, as they may use a wide-angle lens viewing a general area such as a forecourt.
Internet Connection
Your internet connection speed does not need to be fast. It needs to be reliable with an upload speed of about 600kb per second. We only need to send alerts and small face images, not video.
Minimum Technical Specification for Imagus Facial Recognition Server
The minimum specification is as follows. (Note: do not use a less well-specified machine for 4 cameras; an upgrade would be required for more than four(4) cameras.)
(1) The configuration matrix is based on the number of camera streams,
which in terms depends heavily on the video's resolution, frame rate, FOV, and motion.
Cameras
• Recognized high-end cameras, Dome or Bullet style, 2 MP IR camera as a minimum. No auto iris and preferably with a varifocal lens 2.8 to 12mm, so adjustments can be made if needed. A PTZ is preferred for the initial setup, so the pan, tilt, and zoom pan can be tuned.
• Ability to combat external glare - for example, this may help with an entry door camera when considering sunlight or a lot of concrete reflective glare.
• Ability to combat dark conditions, such as a gate in an area with low lighting levels.
• Ideal bandwidth settings 4 MB+.
Angles for best results
Below figure shows the recommended angles for best facial recognition and video analytics results:
• Red Area – 50⁰+ - Bad results
• Orange – 35⁰ - 50⁰ - Varied Results
• Green – 0⁰ - 35⁰ - Best results
Resolutions
• Higher Megapixels cameras such as 4/5K cameras usually have the same sensor size as 1080p cameras, so they do not necessarily have better quality but have more pixel in the same sized sensor.
• Our Imagus Facial Recognition engine performs well with just 32 pixels between the eyes, so it does not need a higher-megapixels camera (i.e., 4/5K), which also comes with a higher price tag.
• 4/5K cameras are more suited for a scenario where there is a requirement to capture faces very far away from the Camera, but it is still possible to use a zoom lens to achieve the same facial recognition and video analytics result with 1080p cameras.
• From a pricing perspective, it's advantageous to recommend 1080p because it uses ¼ of the hardware compared to a higher-end camera.
Frame rates per second (fps)
• The recommended video framerate that the Camera is running depends heavily on the environment and the client's use case.
• In a scenario where we are capturing faces for people coming from a chokepoint, it is essential to know how fast they are moving, e.g., walking or running.
• The average walking speed is 3 to 4 miles per hour, or 1 mile every 15 to 20 minutes. How fast your subjects are moving is a deciding factor on what frame rates are best suited for facial recognition in your use case. As a general guideline, normal walking is ~ 8fps and running is ~ 25 fps.
Target frame rates per second (fps)
• The Target framerate is the Maximum Sample Rate at which we aim to run the facial recognition face detectors.
• This setting is used to limit the amount of GPU used when processing streams.
• Many cameras can send video at higher framerates than the GPU face detectors can process in real-time, especially when processing multiple streams.
• Therefore, to optimise GPU usage, we only want to run the intensive processing detectors on fewer frames and use a lighter tracking algorithm on the intermediate frames. This is a better solution than dropping the framerate of the Camera as we still use the intermediate frames; the more frames that pass through our system, the better the tracking, and the more chances the software has of capturing a good face image for facial recognition.
• The system default target fps is 30.
• The recommended target fps is 12. We would recommend only going lower if there is a need to push the hardware to manage more video streams.
Video Profiles
• The minimum image requirements as follows:
• Sharp image
• Low-resolution faces, minimum ~16 pixels between the eyes, ideal ~50 pixels between eyes
• Grayscale and colour image support
• Formats supported include but are not limited to JPEG, PNG, WebP, H264, H265, MJPG, MPEG4
• It is recommended that any compression option be disabled or minimized (i.e., 0 -10%) where possible to reduce noise for best facial recognition results.
Figure 6 - Same camera, same resolution, same number of pixels (16)
between the eyes 2MB bandwidth
Figure 7 - Same camera, same resolution, same number of pixels (40)
between the eyes 2MB bandwidth
Figure 8 - Same camera, same resolution, same number of pixels (16)
between the eyes 8MB bandwidth
Figure 9 - Same camera, same resolution, same number of pixels (40)
between the eyes 8MB bandwidth
• We do not recommend using compressions such as zip streams or VIQS. However, H264 or H265, when reducing the compression rate down, is acceptable.
Vix Vizion Team










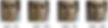

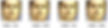
